The Connors Center for Women’s Health and Gender Biology is pleased to work with Mass General Brigham to fund our Annual Connors BWH-MGB Collaborative IGNITE Award. This award builds on the existing IGNITE Awards program that began in 2019.
Investigators from Brigham and Women’s Hospital are expected to collaborate with an investigator from another MGB institution on a joint project. The BWH Principal Investigator (PI) will receive up to $100,000 ($50,000 maximum to the BWH PI and $50,000 maximum to the co-PI at the partnering other MGB institution). The Connors BWH-MGB Collaborative IGNITE Award PI and co-PI will be integrated into the overall Connors Center IGNITE Awards program.
The application cycle for this award is currently closed. Please check back later for the next cycle’s dates.
To access the full RFP and other application materials, please visit our funding opportunities page.

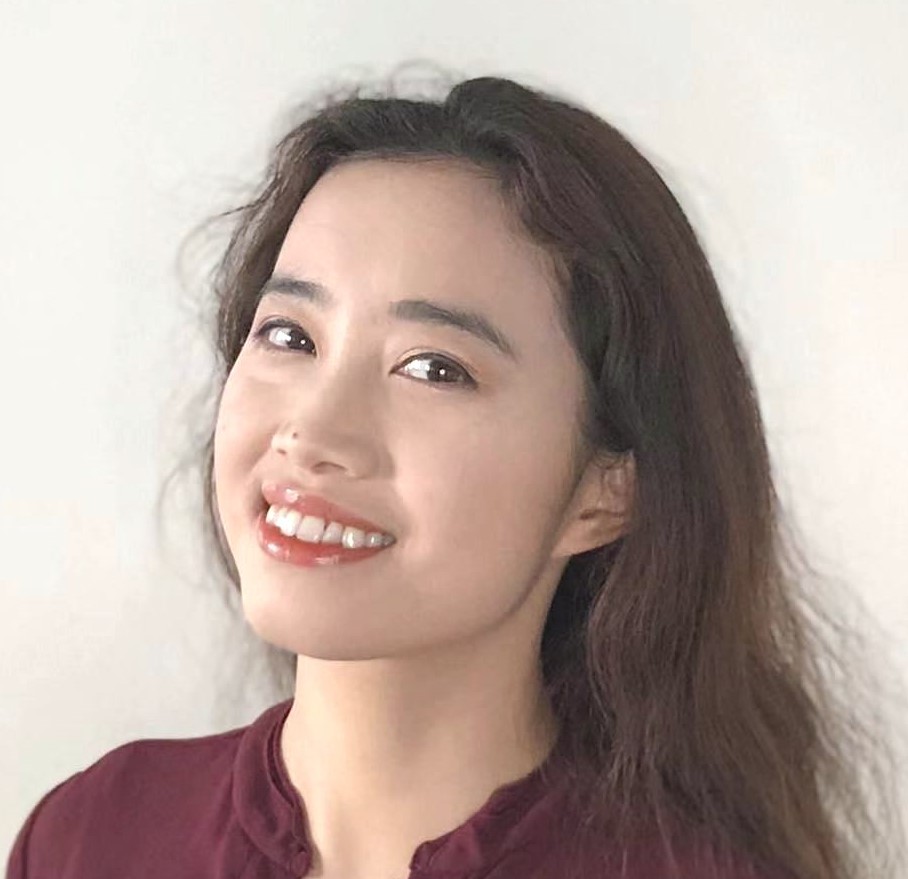
Fei Liu, PhD and Menglu Yang, MD, PhD
2024 Connors BWH-MGB Collaborative Cardiovascular IGNITE Awardees
Department of Medicine, BWH and Schepens Eye Research Institute, MEEI respectively
“Finding New Clues for Sjögren’s Syndrome: Investigating DNA in Tears for Better Diagnosis”
Sjögren’s syndrome, an autoimmune disease affecting tear and saliva glands, affects 4 million Americans, 90% of whom are women. Current treatment options are limited and do not target the root cause of the disease. Prior research has shown Sjögren’s syndrome is associated with internal sensing of DNA, such that tiny particles called exosomes might trigger inflammatory processes. Using the EXODUS method to isolate exosomes from body fluids, this study aims to examine the DNA carried by exosome in the blood and tears of individuals with and without Sjögren’s syndrome, in order to identify differences in DNA carried by exosomes between health and disease. Additionally, mechanisms through which exosome DNA can trigger inflammation in the tear glands will be examined. The ultimate goal is to translate tear exosome biomarkers into clinical applications, thereby benefiting women’s health.
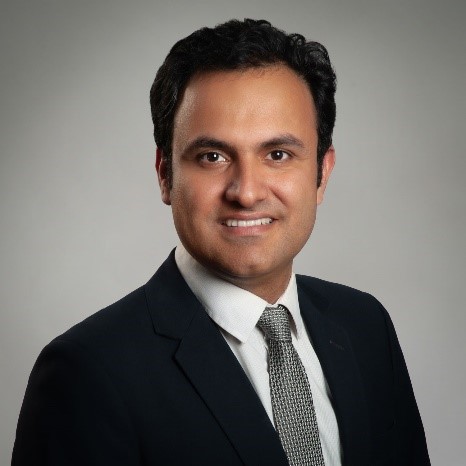
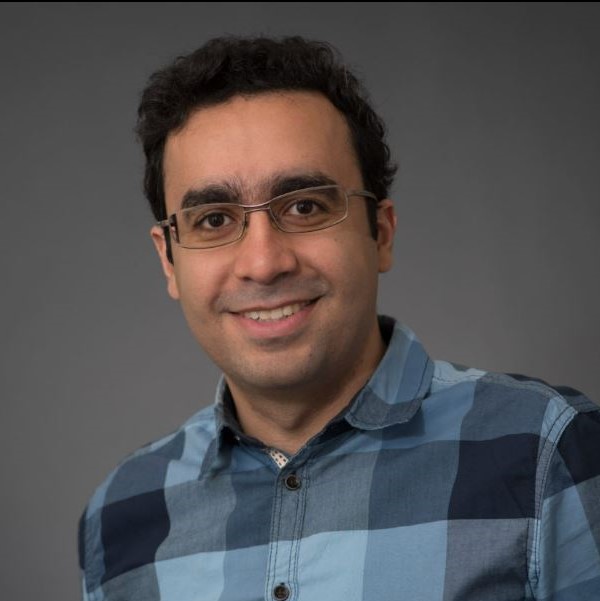
Farhad Nezami, PhD, Iman Aganj, PhD
2023 Connors BWH-MGB Collaborative Cardiovascular IGNITE Awardees
Division of Cardiac Surgery, BWH and Athinoula A. Martinos Center for Biomedical Imaging, MGH respectively
“Leveraging AI Tools for Prediction of Cardiac Remodeling to Enhance Diagnosis and Therapy in Women with Severe Aortic Stenosis”
Women with severe aortic stenosis, i.e. narrowing of aortic valve opening, are often diagnosed at later ages, and reported to be undertreated with excess mortality versus men. Drs. Nezami and Aganj propose an artificial intelligence (AI) powered, mathematical approach that strives to eliminate such imbalances with focus on left ventricle sex-specific shape analysis to ensure equivalent care for all patients with severe aortic stenosis.
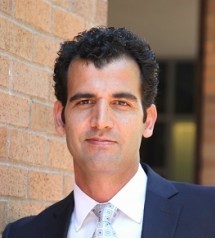
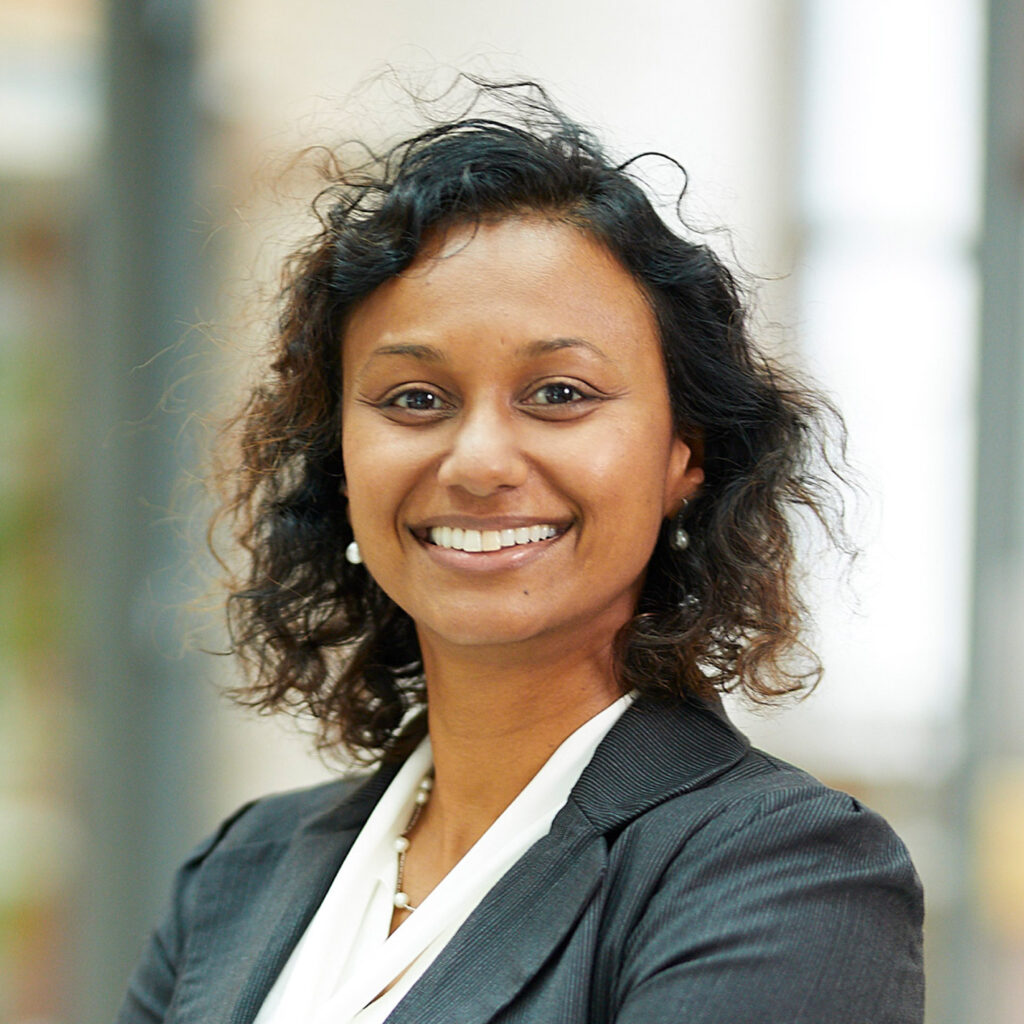
Hadi Shafiee, PhD and Shruthi Mahalingaiah, MD, MS
2022 Connors BWH-MGB Collaborative IGNITE Awardees
Division of Engineering in Medicine, BWH and Department of OBGYN, MGH respectively
“A personalized smartphone-based assay for at-home ovulation prediction in women including those with polycystic ovarian syndrome (PCOS)“
Drs. Shafiee and Mahalingaiah will develop a smartphone-based salivary test, leveraging artificial intelligence, for ovulation detection and tracking for women, especially those with polycystic ovary syndrome (PCOS). The goal of this project is to facilitate fertility planning with a reliable at-home ovulation test.
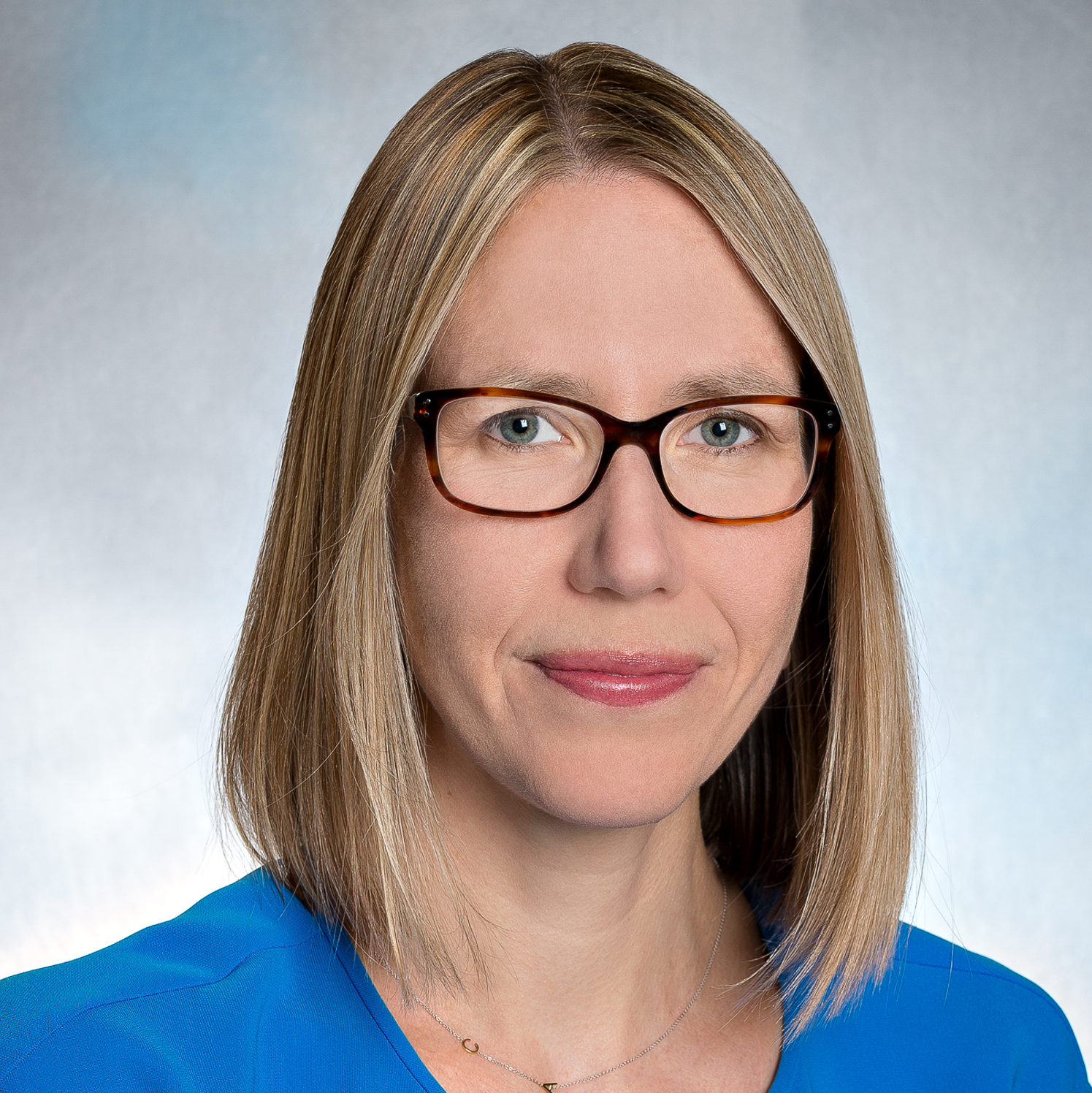
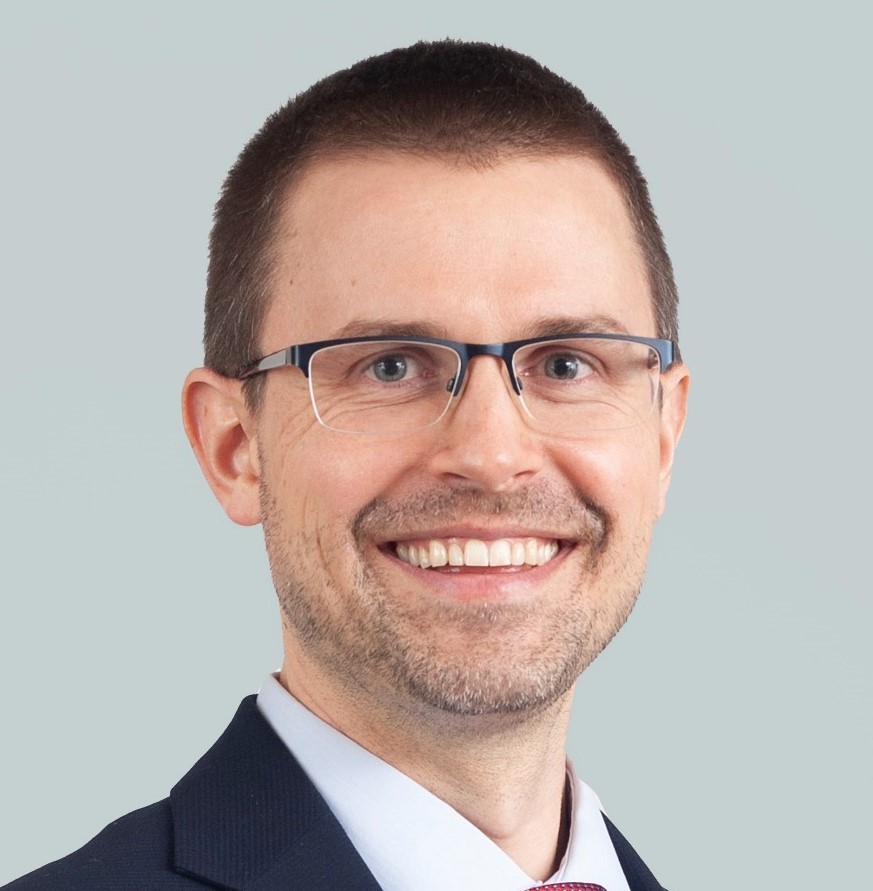
Lydia Pace, MD, MPH and Florian J. Fintelmann, MD
2022 Connors BWH-MGB Collaborative IGNITE Awardees
Division of Women’s Health, BWH and Department of Radiology, MGH respectively
“Tackling sex-differentiating factors to improve lung cancer screening for women: Leveraging machine learning for risk stratification on low-dose chest computed tomography“
Important sex differences exist in lung cancer, but women remain underrepresented in lung cancer research. In this project, Drs. Pace and Fintelmann will utilize machine learning to estimate individuals’ lung cancer risk based on images from CT scans performed for lung cancer screening, evaluate how the accuracy of this assessment may vary by sex, and optimize the machine learning algorithms to improve their performance for both males and females.